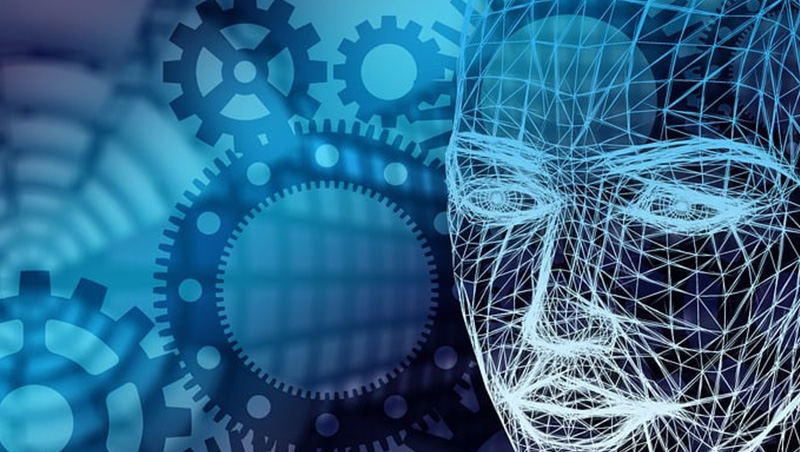
In today’s data-driven world, Spend Analytics has become an essential tool for businesses to gain visibility into their spend and make informed business decisions. At its core, Spend Analytics is the process of analyzing an organization’s spending patterns to identify opportunities for cost reduction, efficiency improvement, and better decision-making. This process has long been a collaboration between automation and human intervention. Creating accurate and usable spend analytics through the appropriately balanced relationship of man and machine is a must.
In prior experience leading a spend analytics group in a big data company I was often integrating automated and human analytic output to bring forward the best results. I’ll mention a few common examples:
Inaccurate Initial Classification: The Human Factor
One of the major challenges we faced was the issue of bad initial spend classification within existing data systems and their associated categorization taxonomies. As we worked on various spend analytics projects, we noticed that client employees claiming to be experts in spend analytics lacked logical classification of the data. Additionally, we observed an overall lack of standardization in spend classification. Different companies and individuals had their own unique ways of classifying spend, often creating an initial baseline of inaccuracy. This inaccuracy became the backbone of ineffective automated analytics. This ineffectiveness of initially established taxonomies, though automated, made it nearly impossible to compare and benchmark data in a meaningful way. It therefore became crucial to establish a standardized and accurate classification system quickly and efficiently.
To evaluate and improve our company’s capability, I suggested conducting pilots on manual Spend data classification based on a sample dataset. We selected Vendor, GL, Material Group, Item Description, and Spend as the basic data fields for the test. And leveraged true category experts to validate results.
The outcome of the pilot test was effective and immediate. The enhanced accuracy highlighted the fact that good Spend Analytics requires iterative work. Spend classification can vary significantly amongst initial taxonomies, often requiring a human validation of initial (and possibly inaccurate) spend classification.
Poor Data Quality: Leveraging Technology to fill human gaps
Another challenge we faced regularly was the quality of data. This was the classic garbage in, garbage out scenario. In many cases, the data we received from our company was incomplete, inconsistent, and often inaccurate. This made analysis difficult and output less than useful.
We had to invest a substantial amount of time in cleaning and structuring the data to ensure its usability. Automation tools such as machine learning and AI proved invaluable in automating data cleaning and classification tasks, providing speed to results and scale that we could have otherwise never achieved.
Conclusion:
Spend Analytics is a critical tool for businesses to gain visibility into their spend and make informed decisions. However, it comes with challenges. Overcoming these challenges requires a combination of experience, expertise, and the use of technologies. The balance of these automated and human process inputs will change over time, but the need for iterative improvement and validation. whether taught by human or automation, never stops. As for now, the relationship remains symbiotic.